top of page
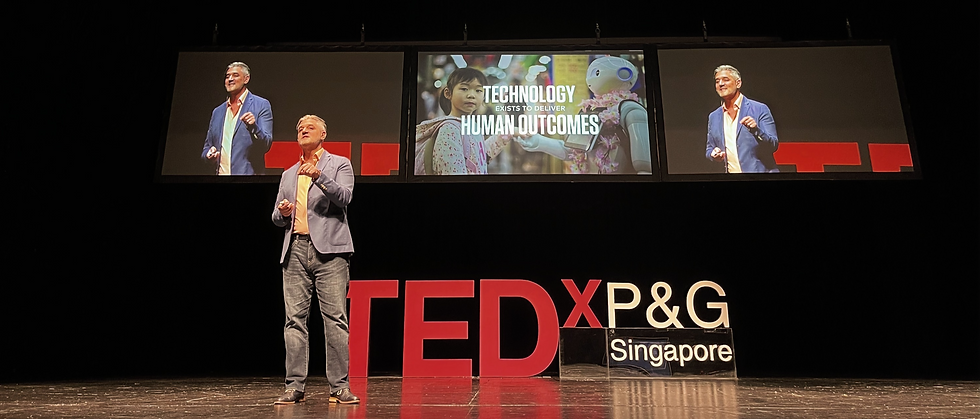
CRA Videos
CRA Videos, Keynote & More
TechBurst Talks Podcast: VIDEO Episodes
TechBurst Talks: One Minute Insights
TechBurst Talks AI: One Minute Insights
TechBurst Talks INNOVATION: One Minute I...
TechBurst Talks IoT: One Minute Insights
TechBurst Talks LIFE: One Minute Insight...
TechBurst Talks TELECOMS: One Minute Ins...
TechBurst Talks VC: One Minute Insights
bottom of page